Model Overview
Model Overview. Our model 𝓜C,z extends CLIP's language input vocabulary with nw novel instance-specific tokens wiy = Clziy, which we model as a linear combination of meta-personalized category features Cl with weights ziy.
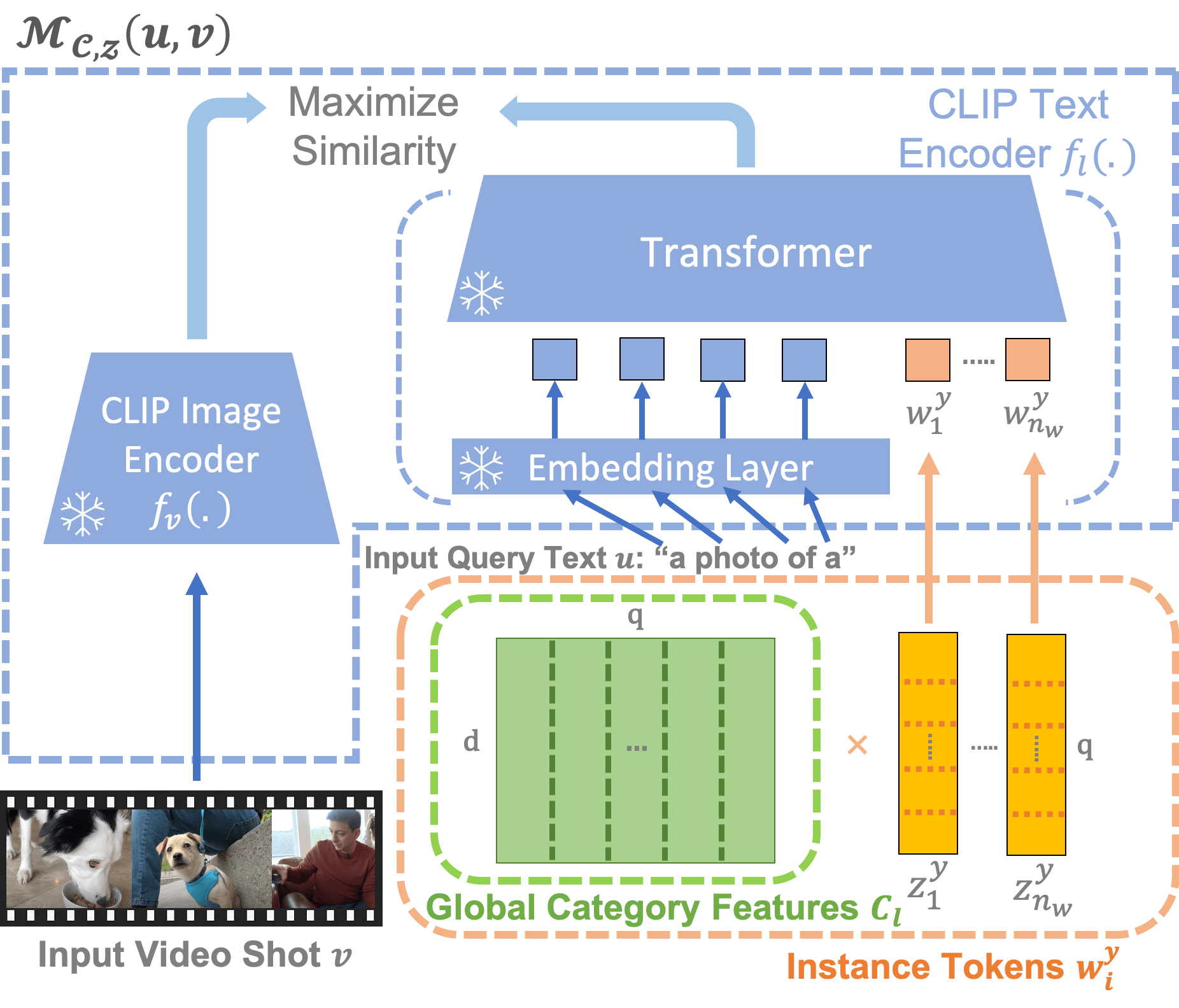
Language Queries | Top-5 Personalized Retrievals |
---|---|
a man is riding <Casey’s boosted board> and wearing white t-shirt and gray shorts |
![]() |
<Zak’s dog Kona> is playing with a black and white dog on the grass |
![]() |
<Zak’s dog Coffee> is lying down in front of a man and three women |
![]() |
<Casey's friend Marlan> is standing on the 2nd floor with a woman |
![]() |
a woman is wearing <Alex's hat> on the grass with black fence behind |
![]() |
Large-scale vision-language models (VLM) have shown impressive results for language-guided search applications. While these models allow category-level queries, they currently struggle with personalized searches for moments in a video where a specific object instance such as ``My dog Biscuit'' appears. We present the following three contributions to address this problem. First, we describe a method to meta-personalize a pre-trained VLM, learning how to learn to personalize a VLM at test time to search in video. Our method extends the VLM's token vocabulary by learning novel word embeddings specific to each instance. To capture only instance-specific features, we represent each instance embedding as a combination of shared and learned global category features. Second, we propose to learn such personalization without explicit human supervision. Our approach automatically identifies moments of named visual instances in video using transcripts and vision-language similarity in the VLM's embedding space. Finally, we introduce This-Is-My, a personal video instance retrieval benchmark. We evaluate our approach on This-Is-My and DeepFashion2 and show that we obtain a 15% relative improvement over the state of the art on the latter dataset.
Personalized Vision-Language Model Overview: Our model enhances a pre-trained VLM (blue) with personalized instance tokens w = Cz (orange), combining global category features C (green) with instance-specific weights z ∈ Z (yellow). Our personalized instance retrieval approach consists of three stages. Firstly, we train global category features CD using automatically collected named personal instances from videos, a process known as Meta-Personalization (left). In the second step (middle), we adapt the meta-personalized category features CD during test-time and learn novel instance weights z ∈ ZP to represent user-specific instances, expressed as w = CPz. Finally (right), we utilize the (frozen) personalized instance tokens w in natural language queries during retrieval.
Model Overview. Our model 𝓜C,z extends CLIP's language input vocabulary with nw novel instance-specific tokens wiy = Clziy, which we model as a linear combination of meta-personalized category features Cl with weights ziy.
Step 1: finds named instances via string-matching of possessive patterns in video transcripts. Step 2: filters non-visual instances using text-to-visual relevance between the instance name and the neighboring shots. Step 3: retrieves additional shots with high visual similarity to the instance reference shot.
Examples from This-Is-My { Meta-Personalization; (top) vs Test-time personalization; (bottom-left) vs Query-time; (bottom-right)} datasets. In the Query-time dataset (bottom-right), we design a challenging video instance retrieval task.
@inproceedings{yeh2023meta,
title={Meta-Personalizing Vision-Language Models To Find Named Instances in Video},
author={Yeh, Chun-Hsiao and Russell, Bryan and Sivic, Josef and Heilbron, Fabian Caba and Jenni, Simon},
booktitle={Proceedings of the IEEE/CVF Conference on Computer Vision and Pattern Recognition},
pages={19123--19132},
year={2023}
}